Curiosities, Anomalies, and Breakdowns in Generative Imagery

Artificial intelligence is emerging as a new revolution in digital image production, opening up unprecedented possibilities for artists and visual creators. Capable of generating visuals with remarkable precision and aesthetic diversity, AI is pushing the boundaries of photographic realism. By disrupting our relationship with images, it also raises questions about authenticity and fuels debates on truthfulness and visual manipulation.
Yet, behind the apparent ease of producing convincing images lies a more complex reality. Achieving a result that aligns with one’s intent often requires numerous iterations, and between each “successful” generation, a stream of strange, failed, or unexpected images accumulates. AI does not always produce what the user expects, and certain recurring anomalies both intrigue and fascinate.
As someone who has encountered these anomalies in my explorations of generative imagery, I find it important to address them. While such images often remain buried in artists’ archives, they reveal something essential about the inner workings of these technologies: their limitations, biases, and their enigmatic, sometimes unsettling nature.
Why Does AI Generate Strange or Incoherent Images?
Unlike humans, who perceive an image as a whole and intuitively understand the relationships between forms, AI relies on a statistical analysis of pixels and patterns it has learned to recognize from its vast training dataset. It does not “understand” anatomy, perspective, or spatial logic as we do—it reproduces them based on correlations it establishes from its training data.
Despite ongoing improvements in generative imaging tools, imperfections remain common. Anatomical errors—such as hands with too many fingers, asymmetrical faces, or impossible postures—occur when the machine attempts to assemble fragments of visual information without a true understanding of the physical characteristics of a body.
More disturbing inconsistencies can also emerge. Sometimes, human figures appear in positions suggesting murder, agony, falling, or dismemberment, even when no element of the prompt seemed to lead in that direction.
If we contextualize these distortions within art history, they recall the trial-and-error processes of artists across centuries. From the Middle Ages, when perspective was not yet mastered and bodies adhered more to symbolic conventions than realism, to the anamorphoses of the Renaissance or the deliberate distortions of Cubism and Surrealism, each era has produced its own forms of visual deformation.
However, while human artists often sought to conceal their mistakes or break conventions through conscious aesthetic choices, AI seems to amplify its errors, as if these distortions were an intrinsic part of its visual language. Why do these patterns appear? Are they mere statistical accidents, or do they reflect a deeper tendency within the datasets used to train these models?
When the Machine “Overflows”
Despite censorship and moderation mechanisms embedded in models like MidJourney or DALL·E, disturbing images continue to emerge. Bizarre scenes, compositions evoking horror or absurdity, dark or surreal atmospheres materialize regardless of the user’s intentions.
These “slippages” are not signs of AI having its own will but rather the result of several underlying factors, such as:
-
Biases in training datasets: The images used to train these models come from the internet, where countless ambiguous or dark scenes exist and can influence outcomes. As Nathan Noiry points out (source), discrepancies between training data and target data can lead to representational biases.
-
Uncertain statistical interpretations: AI does not understand the meaning of the images it generates; it simply associates forms and patterns based on probability. Sometimes, these correlations result in unintentionally disturbing compositions.
-
The limitations of filtering algorithms: While AI models are trained to avoid certain content, they lack a deep semantic understanding, and some images slip through moderation. A European Parliament report highlights that filtering is not limited to illegal content and that arbitrary decisions can occur (source).
A parallel could be drawn with the history of artistic mistakes and missteps: the difference is that AI does not “correct” its deviations as a Renaissance painter would, aware of their own approximations. Instead, it generates, accumulates, and amplifies these anomalies in a process where aesthetic intention is absent.
What Do These Anomalies Reveal About the Data That Shapes Our Humanity?
Beneath the apparent idealism of generative images—their goal to captivate or entertain—lies a lesser-known, more confidential side. These recurring anomalies—distorted faces, grotesque postures, unsettling atmospheres—seem to unveil a deeper truth: the state of our data. Beyond visual refinement, what is at stake here is not just algorithmic errors but a reflection of the fractures embedded within the vast digital archives feeding AI.
If AI is trained on our images, words, and shared behaviors, it is worth questioning what they reveal about us. Do these distortions, these excesses of darkness and disfigurement, serve as indicators of a digital world saturated with biases, gaps, and tensions? In the overflow of collected and shared data, AI sometimes appears to capture the anxieties and hidden shadows of our societies. What seemed like an ideal creative tool thus becomes a sensitive surface upon which, perhaps unintentionally, the concerns, imbalances, and deviations of our time are projected.
These anomalies are not merely glitches—they are symptoms of an era that feeds on its own excess of information, on a relentless production of data often detached from human realities. They remind us that beyond the aesthetic pleasure or intellectual curiosity that AI generates, these images also carry another, more hidden and unsettling truth. They are a mirror of our humanity, in all its complexity and contradictions.
What Should We Do With These flaws ?
Rather than dismissing these imperfections as mere failures, they can be considered an aesthetic approach in their own right. They provide fertile ground for questioning how the machine constructs an image, how it "perceives" our world, and what we define as anomalies: what do they reveal about our own gaze and our relationship to self-censorship? In other words, what are we unwilling to see in ourselves within the distortions and deviations that AI presents to us?
Instead of being relegated to invisibility, these anomalies could be seen as clues, as fragments of a digital memory that escapes our control. For artists and researchers, they open up a space for reflection and experimentation, allowing us to examine what AI reveals—despite itself—about the way we archive, classify, and prioritize representations of the world. Thus, they are not merely accidents but perhaps significant traces of a collective imagery in flux.
-
View the series The progress
-
View more articles
-
Home
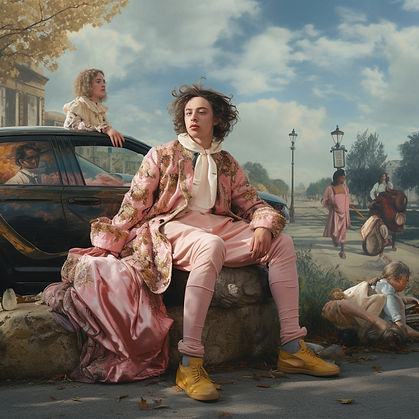